Pay and earnings
Overview
Data source: Produced by the Institute for Employment Research, University of Warwick
Provision: Detailed estimates based on econometric analysis of Labour Force Survey (LFS) and Annual Survey of Hours and Earnings (ASHE) data, constrained to match Office for National Statistics (ONS) published headline figures.
Update: annual
Questions you can ask of the data:
- What is the weekly pay in a particular occupation?
- How much does a 30 year old earn in a particular occupation?
- How many hours do people work in a particular occupation?
Example of visualised data:
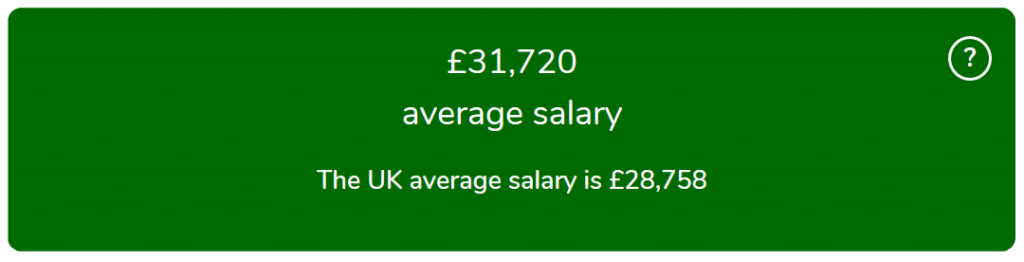
Source: icould
Description of the dataset and provenance
Information on weekly pay (average, median and decile) is taken from a combination of two sources: the ASHE; and the LFS (both conducted by the ONS). ASHE is widely regarded as the most reliable source of information on Pay and Hours, however it does not include
information on pay by qualification as well as some other characteristics (such as self-employment). This information is available in the LFS.
Details of the owner / curator
Although the ASHE data set is based on a relatively large sample, this is not large enough to produce reliable data at the level of detail ideally required. There are also concerns about information being disclosive. Similar problem apply to the LFS. To avoid these problems the raw survey data are not used. Instead a set of estimates have been prepared on behalf of the Department for Education by the Warwick Institute for Employment Research based on the available information and constrained to match published figures. The estimates are based on published ASHE data and the publically available version of the LFS. However, the estimates presented in the database are predictions from an econometric analysis rather than the raw survey results. This avoids problems of breach of confidentiality and disclosure. The results are constrained to match the published totals using an iterative RAS process. The main predictions are made using a standard “Mincerian” earnings equation.
The characteristics of the groups concerned distinguish:
- Gender
- Industry (75 almost 2 digit 2007 classification categories)
- Qualifications (highest held, 9 NQF categories)
- Region/Country (4 UK countries and 9 English regions)
- Age (aggregated groups)
- Occupation (SOC2010 4 digit categories)
Known quality issues with data
Both ASHE and the LFS provide robust estimates, but these are subject to sampling errors when sample sizes are small. Although estimates are currently also provided for 2013, 2015 and 2017, estimates of changes based on comparison between any two years are not statistically robust. For those interested in changes in pay over time a separate “change in pay” indicator is provided, but this is only available for detailed occupational categories cross classified by UK Country or English region. Cross classification by other dimensions (such as industry) are NOT available.
Quality control processes
The API suppresses sample cells with zero or small sample sizes.
Accuracy of data
Precise confidence intervals are not provided around the point estimates. Based on guidelines produced by ONS for general use of LFS data the following “rules of thumb” have been adopted:
- If the numbers employed in a particular category / cell (defined by the 12 regions, gender, status, occupation, qualification and industry (75 categories)) are below 1,000 then a query about the related Weekly Pay will return “no reliable data available” and offer to go up a level of aggregation across one or more of the main dimensions (e.g. UK rather than region,
some aggregation of industries rather than the 75 level, or SOC 2 digit rather than 4 digit). - If the numbers employed in a particular category / cell (defined as in 1.) are between 1,000 and 10,000 then a query on the Weekly Pay will return the estimated figure but with a flag to say that this is based on a relatively small sample size and if the user requires more robust estimates they should go up a level of aggregation across one or more of the main dimensions (as in 1).
- Rounding of estimates – in order to avoid false impressions of precision the API rounds up the estimates before delivering the answer to any query. In the case of the Weekly Pay estimates they are rounded to the nearest £10.
Frequency of update
Does the data underlying the API change over time?
ASHE is conducted on an annual basis (although the LFS is carried out more frequently). The data are updated annually.
Will the data go out of date?
The data are as accurate as they can be at the time they are produced.
Does the data you capture change on at least a daily basis?
No – see above.
What type of dataset series is this?
Information based on a cross-sectional survey of employers (ASHE and LFS).
Is a feed of changes made available?
No, see above.
How frequently do you create a new release?
Annually
What is the delay between creating a dataset and publishing it?
Once the data have been processed they can be uploaded to the LMI for All database.
Do you also provide dumps of the dataset?
No
Will the data be corrected if they contain errors?
Yes
Disclosure and confidentiality
The Department for Education complies with all applicable Data Protection laws in the UK.
The Pay and Earnings data included in this database are non-disclosive.